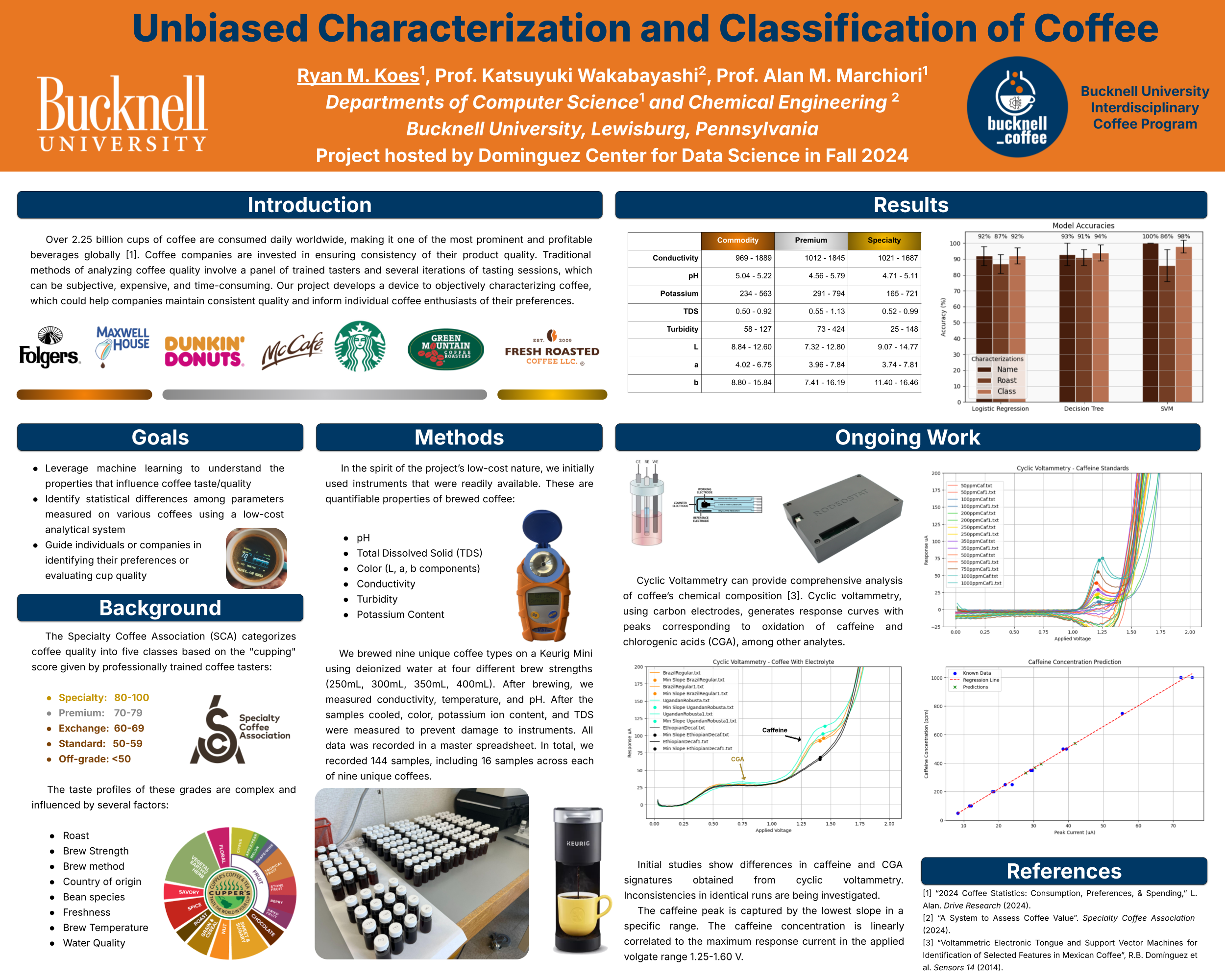
Unbiased Characterization and Classification of Coffee
Author:
Ryan Koes ’26Co-Authors:
Faculty Mentor(s):
Katsuyuki Wakabayashi, Chemical EngineeringAlan Marchiori, Computer Science
Funding Source:
N/AAbstract
Traditional coffee quality assessment relies on certified sensory specialists, who are not easily accessible and can be subjective. These evaluations can lead to inconsistencies in coffee assessment, making it difficult to achieve reliable and standardized evaluations. The goal of this study is to leverage machine learning to objectively classify brewed coffee by brand, class, and roast level using physicochemical characterization. We studied a total of 144 brewed coffee samples from seven different coffee brands ranging across commodity, specialty, and premium classifications, at varying roast levels. For each sample, we measured: pH, color, turbidity, potassium, and total dissolved solids and manually labeled the brand, class, and roast level. We evaluated support vector machine (SVM), decision tree, and logistic regression models to predict coffee brand, class, and roast level. The SVM performed best with 100% accuracy using 10-fold cross-validation.
Our ongoing research explores the use of cyclic voltammetry to analyze electrochemical properties of coffee. This work aims to develop a low-cost electrochemical analysis method to determine distinct characteristics of coffee, providing an objective alternative to traditional sensory evaluation. A screen-printed electrode can detect oxidation peaks of key coffee compounds. Our initial studies are focusing on estimating caffeine concentration. Features of the cyclic voltammetry curve are extracted and used to estimate the caffeine concentration, using High-Performance Liquid Chromatography to measure the ground-truth concentration. Further analysis will extend this approach to include chlorogenic acids, a major class of coffee components, to determine their electrochemical signatures.